Advances in Deep Learning Towards Fire Emergency Application : Novel Architectures, Techniques and Applications of Neural Networks
Doctoral thesis
Published version
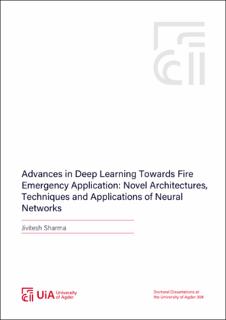
Permanent lenke
https://hdl.handle.net/11250/2721827Utgivelsesdato
2020Metadata
Vis full innførselSamlinger
Originalversjon
Sharma, J. (2020). Advances in Deep Learning Towards Fire Emergency Application: Novel Architectures, Techniques and Applications of Neural Networks (Doctoral thesis). University of Agder, Kristiansand.Sammendrag
Deep Learning has been successfully used in various applications, and recently, there has been an increasing interest in applying deep learning in emergency management. However, there are still many significant challenges that limit the use of deep learning in the latter application domain. In this thesis, we address some of these challenges and propose novel deep learning methods and architectures.
The challenges we address fall in these three areas of emergency management: Detection of the emergency (fire), Analysis of the situation without human intervention and finally Evacuation Planning. In this thesis, we have used computer vision tasks of image classification and semantic segmentation, as well as sound recognition, for detection and analysis. For evacuation planning, we have used deep reinforcement learning.
Beskrivelse
Består av
Paper I: Sharma, J., Granmo, O.-C., Goodwin, M. & Fidje, J. T. (2017). Deep Convolutional Neural Networks for Fire Detection in Images. In G. Boracchi, L. Iliadis, C. Jayne & A. Likas (Eds.), Engineering Applications of Neural Networks. Communications in Computer and Information, 744, 183-193. Springer. https://doi.org/10.1007/978-3-319-65172-9_16183-193. Author´s accepted manuscript. Full-text is available in AURA as a separate file: .Paper II: Sharma, J., Granmo, O.-C. & Goodwin, M. (2018). Deep CNN-ELM Hybrid Models for Fire Detection in Images. Lecture Notes in Computer Science, 11141, 245-259. https://doi.org/10.1007/978-3-030-01424-7_25. Author´s accepted manuscript. Full-text is available in AURA as a separate file: .
Paper III: Sharma, J., Andersen, P.-A., Granmo, O.-C. & Goodwin, M. (2020). Deep Q-Learning With Q-Matrix Transfer Learning for Novel Fire Evacuation Environment. IEEE Transactions on Systems, Man & Cybernetics: Systems. https://doi.org/10.1109/TSMC.2020.2967936. Author´s accepted manuscript. Full-text is available in AURA as a separate file: .
Paper IV: Sharma, J., Granmo, O.-C. & Goodwin, M. (Forthcoming). Emergency Analysis: Multitask Learning with Deep Convolutional Neural Networks for Fire Emergency Scene Parsing. In International Conference on Industrial, Engineering and Other Applications of Applied Intelligent Systems. Springer. Author´s submitted manuscript. Full-text is not available in AURA as a separate file.
Paper V: Sharma, J., Granmo, O.-C. & Goodwin, M. (2020). Environment Sound Classification using Multiple Feature Channels and Attention based Deep Convolutional Neural Network. Proc. Interspeech, 1186-1190. https://doi.org/10.21437/Interspeech.2020-1303. Author´s accepted manuscript. Full-text is available in AURA as a separate file: .
Paper VI: Sharma, J., Granmo, O.-C. & Goodwin, M. (2020). Emergency detection with Environment Sound using Deep Convolutional Neural Networks. In X. S. Yang, S. Sherratt, N. Dey, A. Joshi (Eds.), Proceedings of Fifth International Congress on Information and Communication Technology. Advances in Intelligent Systems and Computing, 1184, 144-154. Springer. https://doi.org/10.1007/978-981-15-5859-7_14. Author´s accepted manuscript. Full-text is available in AURA as a separate file: .