Machine Learning Tools for Radio Map Estimation in Fading-Impaired Channels
Doctoral thesis
Published version
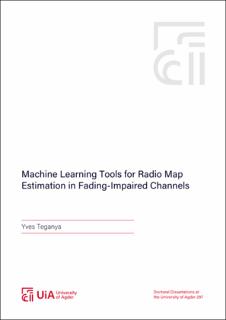
Permanent lenke
https://hdl.handle.net/11250/2687236Utgivelsesdato
2020Metadata
Vis full innførselSamlinger
Originalversjon
Teganya, Y. (2020). Machine Learning Tools for Radio Map Estimation in Fading-Impaired Channels (Doctoral thesis). University of Agder, Kristiansand.Sammendrag
In spectrum cartography, also known as radio map estimation, one constructs maps that provide the value of a given channel metric such as as the received power, power spectral density (PSD), electromagnetic absorption, or channel-gain for every spatial location in the geographic area of interest. The main idea is to deploy sensors and measure the target channel metric at a set of locations and interpolate or extrapolate the measurements. Radio maps nd a myriad of applications in wireless communications such as network planning, interference coordination, power control, spectrum management, resource allocation, handoff optimization, dynamic spectrum access, and cognitive radio. More recently, radio maps have been widely recognized as an enabling technology for unmanned aerial vehicle (UAV) communications because they allow autonomous UAVs to account for communication constraints when planning a mission. Additional use cases include radio tomography and source localization.
Består av
Paper I: Teganya, Y., Lopez-Ramos, L. M.; Romero, D. & Beferull-Lozano, B. (2018). Localization-Free Power Cartography. In 2018 IEEE International Conference on Acoustics, Speech and Signal Processing (ICASSP) (p. 3549-3553). IEEE. https://doi.org/10.1109/ICASSP.2018.8461731. Author´s accepted manuscript. Full-text is available in AURA as a separate file: http://hdl.handle.net/11250/2594807.Paper II: Teganya, Y., Romero, D., Lopez-Ramos, L. M. & Beferull-Lozano, B. (2019). Location-free Spectrum Cartography. IEEE Transactions on Signal Processing, 67(15), 4013-4026. https://doi.org/10.1109/TSP.2019.2923151. Author´s accepted manuscript. Full-text is available in AURA as a separate file: https://hdl.handle.net/11250/2647926.
Paper III: Teganya, Y. & Romero, D. (2020). Data-Driven Spectrum Cartography via Deep Completion Autoencoders. In ICC 2020 - 2020 IEEE International Conference on Communications. https://doi.org/10.1109/ICC40277.2020.9149400. Author´s accepted manuscript. Full-text is available in AURA as a separate file: .
Paper IV: Teganya, Y. & Romero, D. (Forthcoming). Deep Completion Autoencoders for Radio Map Estimation. IEEE Transactions on Wireless Communications. https://arxiv.org/abs/2005.05964. Author´s submitted manuscript. Full-text is not available in AURA as a separate file.